Robots Are Learning To Brace Themselves Against Walls So They Don’t Fall
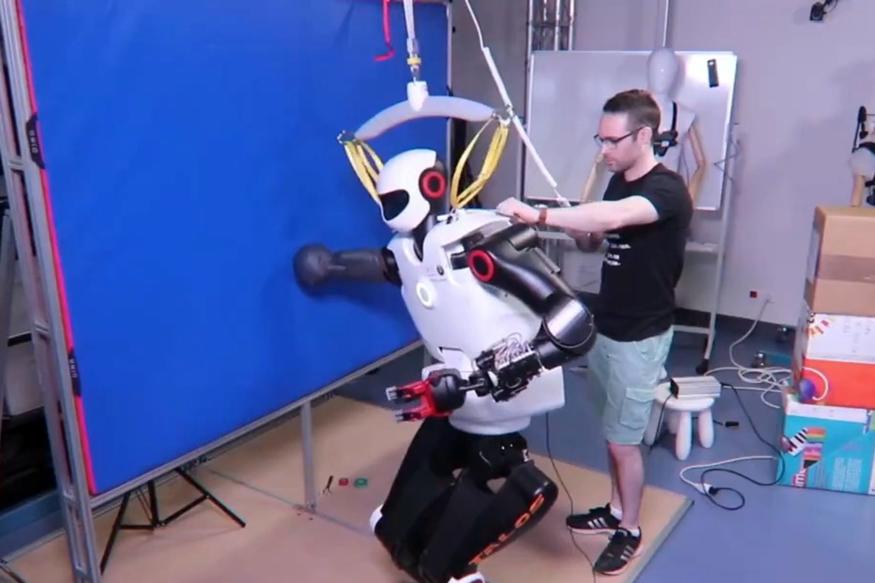
Researchers at the University of Lorraine have developed a “Damage Reflex” (D-Reflex) system in which the humanoid robot TALOS leans against a wall when one of its legs is broken, like a human who has just lost his balance. The neural network based system uses its experience (882,000 training simulations) to quickly find the point on the wall that is most likely to provide stability. The robot doesn’t need information about how it was damaged and can reach out about as fast as a human.
D-Reflex does not guarantee fall prevention as it cannot account for every possible position or surface. It also doesn’t help the robot recover after averting a disaster. The current approach is also based on a stationary bot and won’t help if the drive fails halfway through.
The researchers hope to create a system that is useful on the move, and envision robots that can grab hold of difficult objects when a fall is imminent. This could save the cost of replacing worker robots that are otherwise doomed, and could lead to more “natural” bots that learn to use the environment to their advantage.